What Does a Data Scientist Do?
BrainStation’s Data Scientist career guide can help you take the first steps toward a lucrative career in data science. Read on for an overview of the job responsibilities of a Data Scientist.
Become a Data Scientist
Speak to a Learning Advisor to learn more about how our bootcamps and courses can help you become a Data Scientist.
A Data Scientist is a data expert that extrapolates insights from large data sets to help organizations solve complex problems. To do so, Data Scientists combine computer science, mathematics, statistics, and modeling with a strong understanding of their business and industry to unlock new opportunities and strategies.
Data Scientist Job Description
A Data Scientist’s specific tasks vary greatly depending on the industry they’re in and the company they work for. Generally speaking, though, a Data Scientist job description will usually include all or most of the following roles and responsibilities:
- Researching an industry and company to identify pain points, opportunities for growth, and areas for improvement in efficiency and productivity (among other things).
- Defining which data sets are relevant and useful, then collecting or extracting that data from various sources.
- Cleaning data to remove anything unusable, and testing it to confirm that what remains is accurate and uniform.
- Creating and applying algorithms used to implement automation tools.
- Modeling and analyzing data to identify latent patterns and trends.
- Visualizing data or organizing it into dashboards that other members of the organization can consult.
- Presenting findings and making recommendations to other members of the organization.
A Day in the Life of a Data Scientist
The common perception that a day in the life of a Data Scientist is spent crunching numbers is not too far off the mark. do work with large sets of data, deciding what data is needed, cleaning the data, building models of what the data can show, and organizing it to reveal latent information—and this effort is always directed toward some kind of goal.
Notably, those data sets aren’t always numbers. While most Data Scientists do work with numerical data (73 percent, according to the BrainStation Digital Skills Survey), there are other types of data as well. According to the same survey, 61 percent of respondents work with text, 44 percent with structured data, 13 percent with images, and 12 percent with graphics—even video and audio are ripe for analysis, with 6 and 4 percent (respectively) of respondents working with these media regularly.
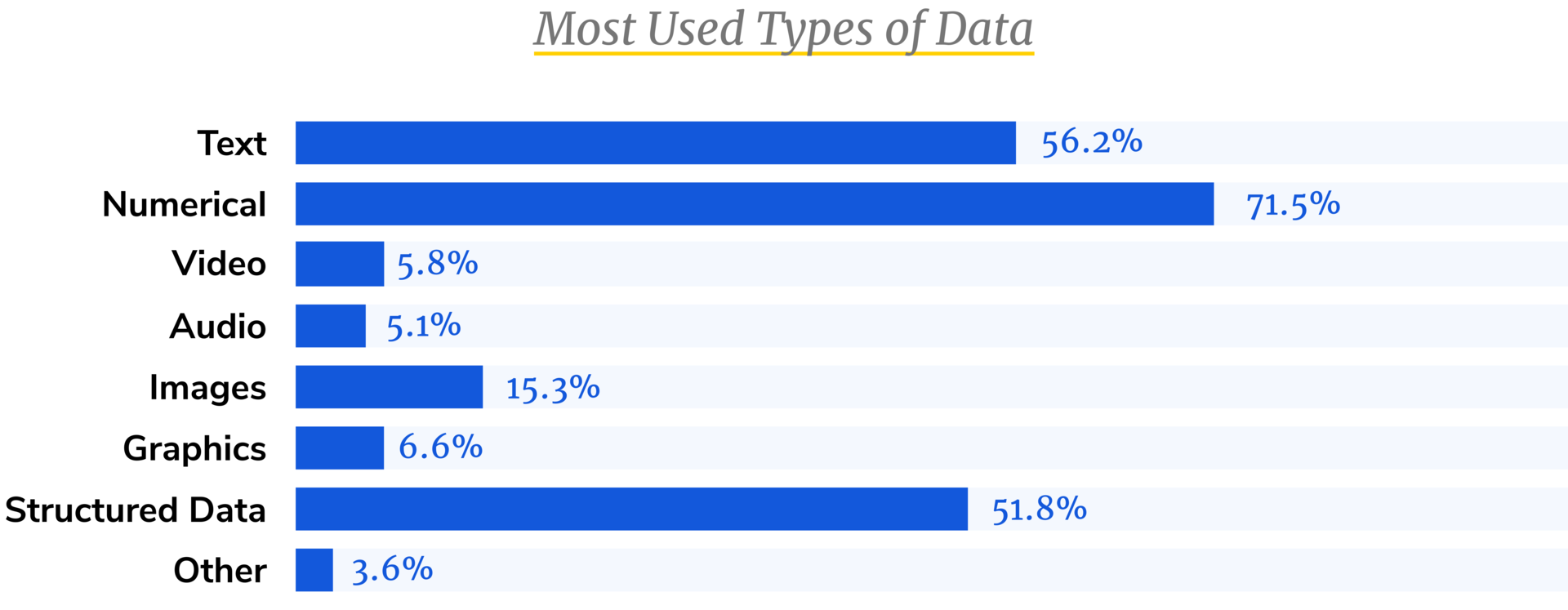
These results hint at the way data science is expanding far beyond the world of financial tables, and exerting its influence in areas like maximizing customer satisfaction and extracting valuable insights from social media.
As a result, every industry has its own types of data, and its own ways to leverage that data to help meet desired outcomes. In every case, though, data science serves as a way to help leadership make better, more informed decisions—whether that’s improving a product, understanding a new market, retaining customers, effectively deploying a labor force, or making better hires.
Data Scientists, therefore, use a combination of techniques and concepts, including:
Descriptive analytics
Studies large sets of data to understand the way things are, including correlations and even causations that aren’t immediately obvious.
Predictive causal analytics
Draws inferences from data using a variety of statistical techniques—including data mining, predictive modeling, and machine learning—to predict the possibilities of a future event.
Prescriptive analytics
Provides intelligence-based recommendations to produce a desired outcome or accelerate the results of a given application or business process.
Machine learning
To put it simply, machine learning – or the process of a computer learning how to better perform a task as it gains more experience doing so – uses algorithms to make predictions and find patterns. Machine learning spans a wide array of ideas, tools, and techniques used by Data Scientists and other professionals, and it’s one of the most popular methods for processing big amounts of raw data.