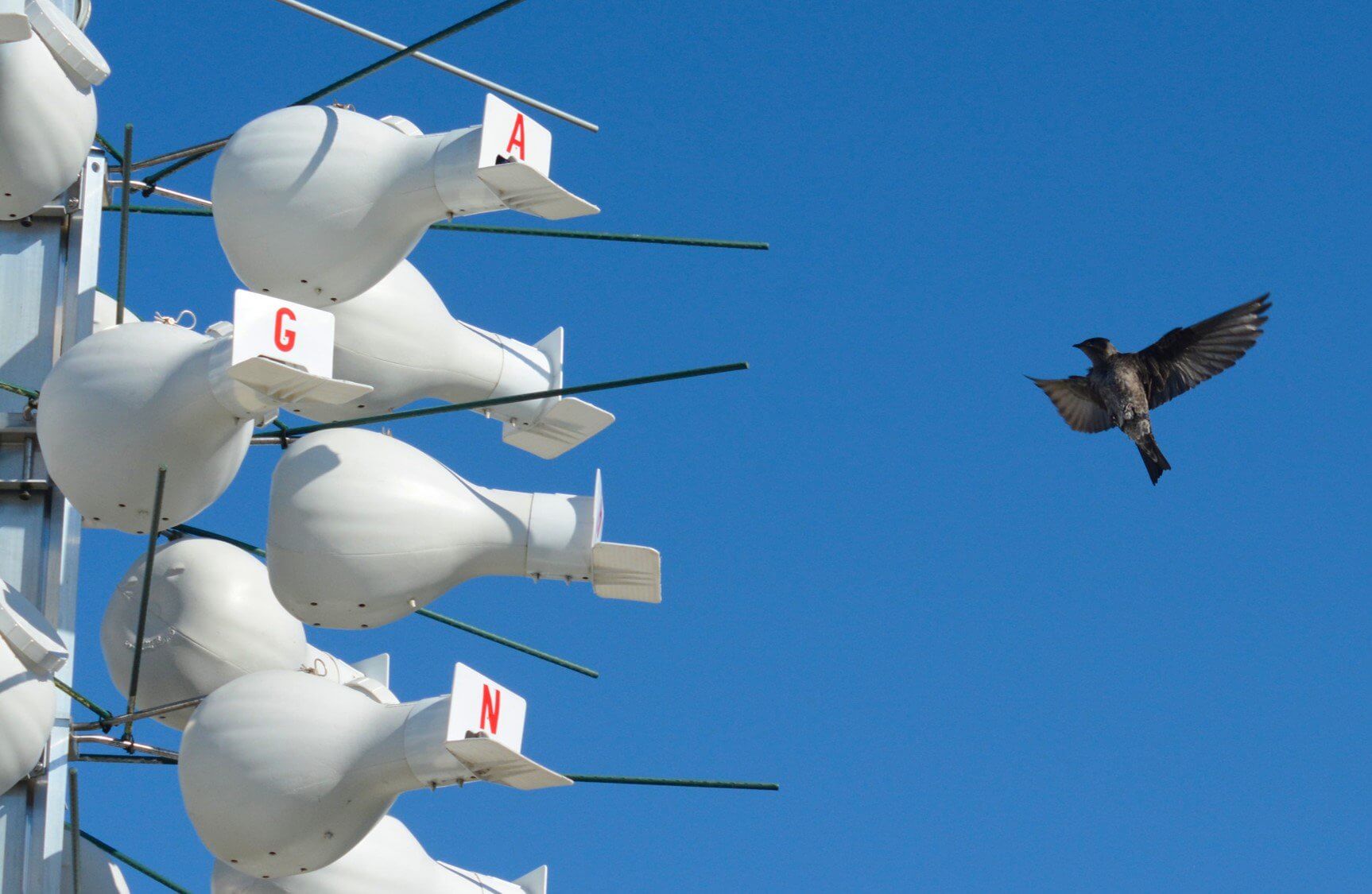
It’s hard to convey just how important environmentalism is. It affects all industries as well as everyone on the planet in one way or another, so it is important to take a comprehensive look at how to fight signs of climate change and impact the world in a positive way.
Microsoft has taken that challenge under their wing with the AI for Earth initiative. The program awards grants to support projects that monitor, model, and ultimately manage Earth’s natural systems. Individuals, groups of students, or organizations can apply for grants, and so far Microsoft has awarded 139 grants spread over 45 countries. The funding comes in the form of Azure grants which allows the projects to access a wide depth of Microsoft resources including machine learning tools and computer vision capabilities.
“What AI for Earth has to be is this kind of platform program,” explains Lucas Joppa, Microsoft’s chief environment officer. “We have the cloud, we have Azure, and we have the services that run on there, so what AI for Earth is all about is providing that next level of services which then empower anybody to take those building blocks and contextualize them to their local problems.”
When it was first launched last year, AI for Earth promised $2 million in total grants. That amount has since been raised to $50 million over the next five years, and Joppa explains that Microsoft will deem the initiative successful if even just a handful of the projects scale up effectively using Azure technology to tackle global problems.
AI for Earth covers four main pillars when it comes to projects and grants: agriculture, water, biodiversity, and climate change. As these four areas are extremely wide and diverse, that means the projects that Microsoft takes in must also represent the diversity of a changing world. That means it cannot simply be solutions for problems facing western countries, or very niche projects applying to only one area of the world.
Below are some of the most interesting projects Microsoft awarded grants to during the first expanded year of AI for Earth.
Fighting rainforest deforestation through smart contracts and gamified blockchain technology (ETH Zurich)
GainForest builds on the idea that those with money riding on something are more likely to be more engaged in that field—for example, someone who has bet on a hockey game will watch it much more intently. In this case, GainForest uses monetary stakes to incentivize people to act as caretakers for at-risk areas of the rainforest. Up to 80 per cent of deforestation is caused by local farmers looking to make room for cattle, so this program uses smart contracts to fight back.
A transparent and scalable platform allows anyone to be a stakeholder by placing money on at-risk areas (identified through a neural network) via a donation. They can then see in real-time as a local caretaker takes responsibility for a patch of the Amazon rainforest, ensuring it does not get demolished. If after a period of six months, the plot remains untouched, the money is given back, plus interest.
In the above image, stakes are placed on four forested areas. As those areas get cleared and destroyed, the stakes diminish. At the end of the period, a largely untouched is rewarded with much more money, while the areas that suffered heavy deforestation either get no money or very little.
“We can use smart contracts to gamify the conservation approach,” says David Dao, a founded member of GainForest. “We’ve had a lot of interest but we want to make sure a pilot can work before we expand it further.”
Predicting crop phenotypes with deep learning (University of Victoria)
At the beginning of every growing season, farmers plan hundreds of seeds to find out which one will be the most desirable for their land and area. This can be a brutally time-consuming process, as they must investigate each plant for several key features and determine which one will yield the most.
This project uses deep learning to identify key traits in desirable plants, all from photos either taken by hand or through a drone, saving huge amounts of time and putting money back into farmer’s hands.
“Having a computer recognize these traits has potential to increase speed, reliability and precision of trait identification and will provide new opportunities for crop breeders and farmers to directly compare large numbers of individual crops with differences in genetics, growing environment and crop management,” said Ian Stavness, a member of the team working on the project.
Cleaning up oceans through bridge cameras equipped computer vision (Southern California Coastal Water Research Project)
The Ocean Cleanup may be a familiar name to some environmentalists, as the organization’s giant ocean ropes are helping to rid the sea of innumerable tonnes of plastic each day. But this newest project looks to address another source of ocean plastic: rivers.
It is estimated that close to 20 per cent of the plastic in the oceans is deposited there by streams or rivers. This project straps cameras onto the undersides of bridges to track what kind of garbage and how much enters an ocean. Deep learning networks identify the trash and send information to the organization, enabling better cleanup efforts and a stronger allocation of resources to target areas.
Learning forest wildfire dynamics with reinforcement learning (University of Waterloo)
Forest fires are a peculiar thing—they can cause widespread damage and destruction, yet they are necessary for some areas in order to burn away the undergrowth and allow the plants and trees to grow and bloom.
Due to this, it can be extremely difficult to track them and learn the habit of a fire before it is too late. This project uses reinforcement learning to track how fires have spread in the past. This allows better prediction of when a fire will hit a populated or important area, and when the fire can be left to burn.

With this technology, the fires that affected Fort McMurray would have been able to be predicted at a much quicker rate and an evacuation could have come much sooner, according to a paper from the project’s leads.
Stopping poaching through a network of cameras armed with computer vision and deep learning (Peace Parks Foundation)
A headline everyone hates is one that involves the illegal poaching of endangered animals. So a team of researchers and environmentalists from Peace Parks in South Africa got together to solve the problem intelligently.
30-plus cameras were installed around a national park in the country, alerting and taking a picture anytime something came across their path. A deep learning algorithm separated photos of humans, and then went one more step to identify those who may be a potential poaching risk. An alert would be sent to local law enforcement and conservationists, and they would dispatch to address the concern—all in around three minutes.
So far, the system has caught several poachers and contributed valuable evidence to apprehend several more. The cameras are completely open to the public, so in the future, they could potentially be used to track migration and mating habits.