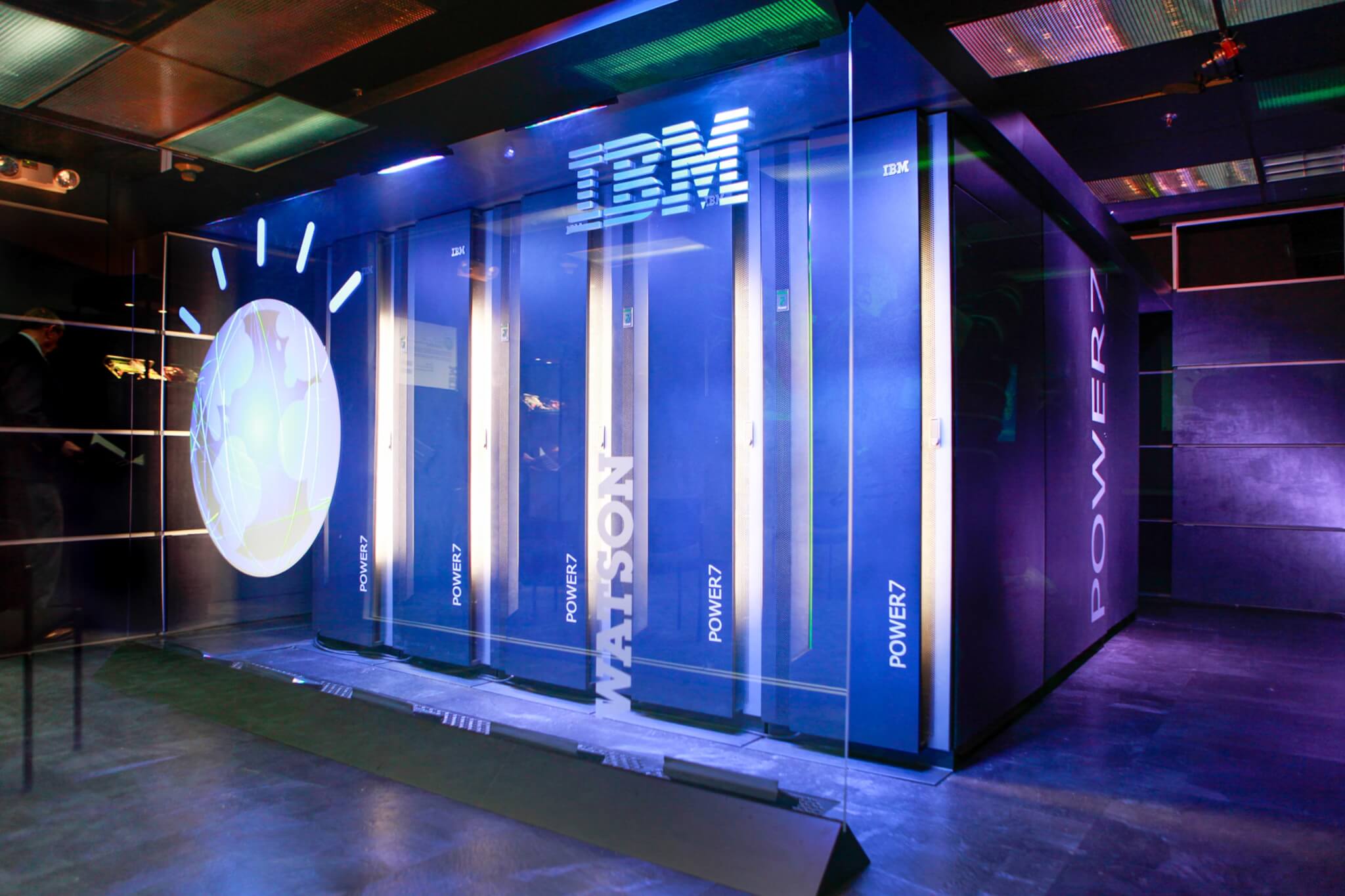
Not all Artificial Intelligence is created equal. Depending on the problem it’s trying to solve, a particular AI solution originates in a few different ways. The average tech-forward firm interested in tapping AI to solve business challenges might create a small, in-house team of data scientists using a combination of custom and tweaked open source code. In other cases, proprietary AI research might even be purchased through a merger or acquisition—an acqui-hire, as it’s sometimes called.
As a leader in AI, IBM has found a middle ground and it’s leading them to some of the most compelling projects ever seen in the space. Just under two years ago, IBM opened the MIT-IBM Watson AI Lab, an industry research organization dedicated to soliciting and creating cutting-edge AI offerings in several different fields such as healthcare, security, and finance.
There is almost always a clear separation between academic research and industry application of emerging technologies, and AI is no different. Academic research is often grounded in theoretical use cases with post-secondary faculty setting timelines of three-plus years to actually witness tangible impact within an industry. And more often than not, it’s longer than that.
Academia is interested in testing the limits of AI research and using those resources to solve problems that the industry is years away from facing; a scenario that creates rifts in a business climate where the competition moves so quickly that being first counts for a lot. With this dynamic, businesses typically stray away from deep academic partnerships and instead simply provide funding with the hope of fostering goodwill. This practice often leads to hiring those researchers rather than creating a patentable and deliverable AI solution.
IBM, however, is investing $240 million USD over 10 years to fund the lab. The tech giant is paying a quarter-billion-dollars to keep some of the smartest people in the world on retainer and build solutions that have the capability to redefine what modern AI looks and like.
“The usual model is that the industry solicits something they want, then academics will respond to the solicitation. The industry will put money over the wall, then for better or worse the institution takes that money, says thank you, and goes off and does whatever they’re going to do,” explains David Cox, the IBM director of the MIT-IBM Watson AI Lab.
While the funding (which comes in the form of grants and donations) is helpful, in practice it amounts to baby steps. Historic advances in enterprise AI rely on academic breakthroughs. Geoffrey Hinton’s historic 1986 research paper ‘Learning representations by back-propagating errors’ invented deep learning (it’s been cited almost 18,000 times), and his 2012 paper ‘ImageNet Classification with Deep Convolutional Neural Networks’ brought machine learning to the masses, enabling companies like Google—who actually snapped up Hinton as a lead researcher for Google Brain—to become the leaders in AI that they are today.
The MIT-IBM lab, as well as the research conducted within, is obviously impressive. As a central meeting place for AI’s top talent (MIT has been ranked as the world’s number one university for the last seven years), the decision to partner is an easy one to make. However, the biggest question revolved around how IBM would walk the fine line between funding academic research and then turn it around to find real enterprise use cases.
Converting AI research to enterprise solutions
As fine a line as it may be, there is a way to maneuver it, and it has to do with the different ways AI projects are conceived. Currently, there are over 3,000 employees working in IBM’s research arm alone, with a large section of data scientists. That IBM division is incredibly apt, with Nobel laureates and Turing award winners walking the halls. But they are creating enterprise solutions that clients can roll out within tight deadlines of under a year: Recognition interfaces, personalization engines, and other features deploy every week, creating streamlined platforms for millions of IBM’s secondary customers. As intelligent as the IBM research team is, they have deadlines and client deliverables academic research teams would never be held to.
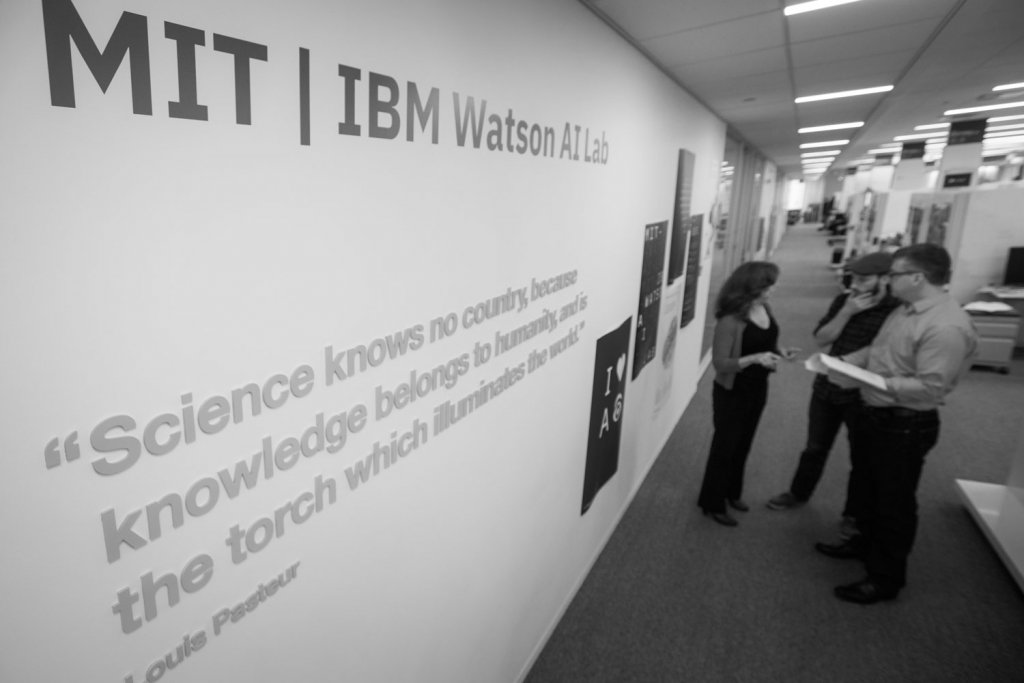
Consider that IBM has thousands of customers, ranging from Fortune 500 companies to small businesses. One thing is common between each client, and it’s that they want solutions that deliver value quickly. Academic research labs don’t normally work on quick value projects, so for a business, the value of an academic partnership is the hiring advantage that stems from goodwill.
“Really what’s happening behind the scenes is that industry is not giving the money so much to get an outcome in terms of technology, but rather to just have an option to hire the students from under the faculty members,” says Cox. “Investments are more relationship building than anything. There’s not a ton of real creation of technology, and that’s reflected in the dollar amounts of industry funding academia. It tends to be smaller amounts with shorter engagements. They’re really just buying goodwill in the research community.”
The extreme end of that spectrum occurred in 2015 when Uber snapped up one-third of Carnegie Mellon’s robotics engineering centre to form Uber Pittsburgh and research autonomous driving. The ride-sharing company had previously partnered with the university, but that goodwill led to 50-plus researchers leaving to become engineers at Uber.
“Because we’re working together at the level of code and not just relationships, we can do a lot more here.” – David Cox, Director, IBM-MIT Watson AI Lab.
Situations similar to Uber’s poaching of research talent is usually what happens when industry and academia form partnerships, though not always to that extent. IBM and MIT carved a new path, largely due to IBM owning a stake in what happens within the lab. They know these solutions might not be rolled out until years down the line, and the ones that are already showing promise look like they might influence entire industries for decades to come.
“This is something different, and I think it’s really important how it’s different,” Cox explains. “IBM research is almost like a university itself. We’re working on projects with MIT together, and that really changes the equation. It means that we have a stake—we don’t have spies. We’re really doing these projects with colleagues. That’s an interesting challenge to make these collaborations happen—they don’t happen by themselves. We need the researchers to find each other from the bottom up then propose what the most interesting problems to work on are.”
IBM itself has strategic goals that must be met. In order to solicit projects for the lab, they launch RFPs. The lab is looking for proposals that will advance AI Algorithms, Physics of AI, Applications of AI and Advancing Shared Prosperity through AI, and award up to $400,000 per year for each project. The first round of proposals launched 48 projects from a pool of 186 applications, and the second call for projects just finished last month.
Problems without solutions
IBM spells out what they think are important problems that move the needle for both the company itself as well as its clients. Then the MIT/IBM researchers—about 100 in total—come together to find the right direction to solve those problems. It is a “really collaborative approach from top to bottom,” according to Cox. This is the step where most companies disconnect from potential enterprise AI solutions. After all, a three-year project that may or may not return a deliverable solution—with the added chance that the solution will still take years to actually launch—is not something most organizations want to be a part of.
The good thing is that when close to 50 projects are being funded and researched, at least a few will demonstrate real-world application within a short timeline, and that window will be seized.
“If we see an opportunity, of course we’re going to take it,” says Cox.
He points to research the lab is currently doing in the realm of neural network architecture, which is the ability to use AI to design AI projects. IBM collaborated with MIT professor Song Han to develop a neural architecture search (NAS) technique that created a convolutional neural network (CNN), which aids in image classification and detection tasks. Before this project, it took 48,000 GPU hours to create a single CNN. Han’s research brought that down to 200 GPU hours.
Cox and other lab members recognized this opportunity immediately and hired one of Han’s students as an intern, which provided him access to IBM’s systems and resources in a much more direct way. Now the lab is working to transition the NAS techniques to become a viable product that may soon be able to roll out within IBM’s enterprise clients.
“It’s an interesting model where academia itself might struggle to see that kind of impact so fast,” says Cox. “But because IBM is right there, we’re already familiar and we have people, and we’re also adjacent to the people who own the product, so we can go ahead and just go straight into that inclusion of the product.”
“We’re working on projects with MIT together, and that really changes the equation. It means that we have a stake—we don’t have spies.” – David Cox
From a business perspective, CNNs really help with rollouts like the application of natural language processing, a massive trend considering how popular voice technology is getting. This research project has the ability to aid any size company in creating its own natural language processors instead of piggybacking onto the currently-available platforms. If more SMEs can experiment with custom-built language processors, the entire industry will move forward like never before.
This is the differentiation between enterprise AI research and academia—one is building a body part, while the other is building a brain.
“Because we’re working together at the level of code and not just relationships, we can do a lot more here,” says Cox. By actively funding long horizon AI goals while also having the wherewithal to understand quickly-actionable research and monetize when need be, IBM’s clients find themselves in a win-win situation.
In the past, Cox has explained it by saying if someone wants to get to the moon, one way to get closer is to climb a mountain. That may technically bring the person nearer to their goal, but it’s not the right path. Focus attention on making the big leap and build a rocket. Advances like the lab’s research into NAS techniques is a rocket, while those traditional AI platforms of today—chatbots and structured image recognition—is climbing the mountain.
“We get customers to guide us on what problems they see, then we can bring the firepower of these really intelligent researchers,” says Cox. “They say ‘well, okay, we’ve looked at this problem, and this is something today’s AI won’t help, but we can invent new AI to break this barrier.’”
For a more practical solution rooted in real-world concern, think of it like this: One-third of food created for human consumption is lost or wasted. How can AI break that barrier? The way the lab works is such that they are not necessarily creating solutions targeted for one enterprise, but instead researching steps forward that will reinvent how companies approach challenges within their industry.
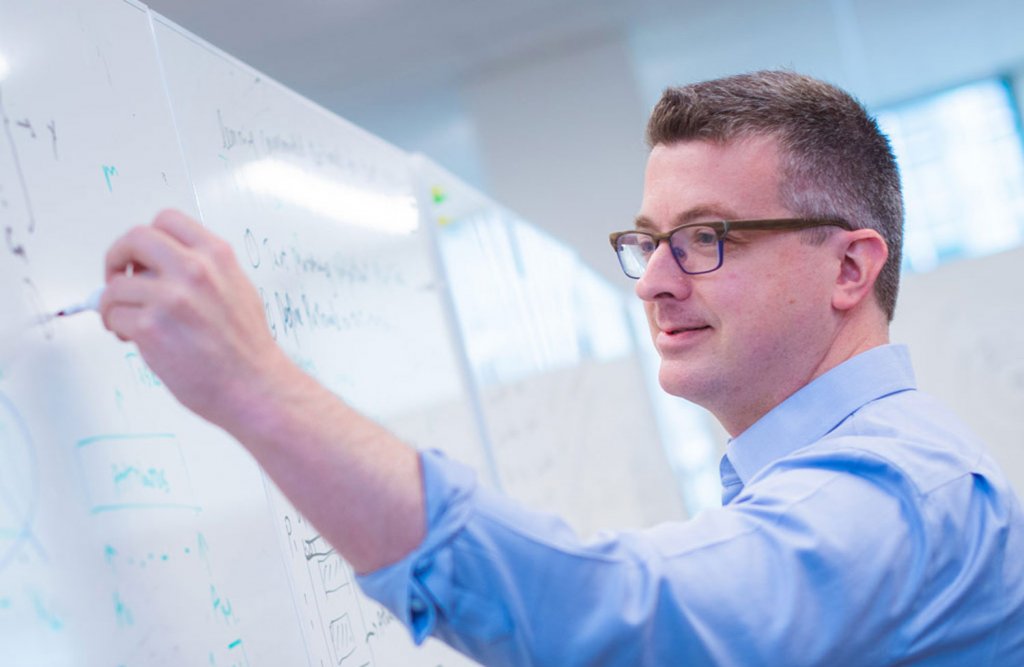
A team at the lab is currently creating a system that builds and tests new structural proteins that will act as edible shields to surround perishable foods, extending their shelf life by an immense period. Using machine learning, researchers are analyzing amino acid sequences that make a protein edible, then using proprietary algorithms to accelerate structural modeling and see how those proteins function in a live environment.
More in line with academic AI, there is no timeline as to when this solution might make it to the shelves. The long game matters here though, as IBM counts massive food retailers like Albertsons and Walmart as clients. Both are already engaging the tech giant in nascent technologies like blockchain inventory management, so the possibility of passing along an AI food preservation solution is very within the realm of possibility.
Scaling beyond enterprise
At any given time, the IBM-MIT lab has dozens of active AI research projects, but the overall goal for the lab is one that moves the needle forward for any size enterprise: Remove the barriers faced when implementing AI.
Investing in AI costs a lot of money and it requires a lot of time along with enormous pools of data to actually train a machine learning platform. For most SMEs, it is simply not worth the investment to create in-house solutions, because, at the very least, they either do not have enough data or cannot structure their existing data to optimize it for current AI solutions. To break this barrier, the lab is working towards a shift from what IBM calls ‘narrow AI’ to ‘broad AI,’ which is the ability for AI to learn from smaller fragmented sets of data.
Even from the perspective of an enterprise with almost-unlimited resources, there are some challenges broad AI will just never be able to solve. Cybersecurity, for example—an algorithm cannot be trained on millions of network intrusions, because the very first one is meaningful, and by the time it is detected, it’s too late. There may be ways around it, but many AI systems will simply never see enough data, or it will be far too expensive to obtain and secure the data.
“Today’s AI is exciting and disruptive, but there’s an awful lot of problems—maybe even the majority of problems—that enterprises have that are not well-served by the kinds of tools that, for instance, deep learning provides,” says Cox.
“A big part of what we are about is lowering those barriers to work on big data and small data. We’re proof that you can work with small data sets. We don’t need to see thousands and millions of examples of something to be able to operate. Modern AI, as powerful as it is, needs a mountain of data to operate.”
Businesses right now operate based off humans who can look at small groups of data and make decisions across several disciplines. This is business 101—the most successful leaders recognize trends and act on them. The lab is working on enabling AI to act in a similar fashion, and when that shift happens, it will be monumental. Recognize trends across different industries and data pools, then make informed decisions applicable to one specific business use-case.
“That will be absolutely disruptive,” Cox says. “As we gradually get to the point where you can operate with the number of examples a human needs rather than the number of examples a supervised deep neural network needs, that will open up a huge range of applications.”