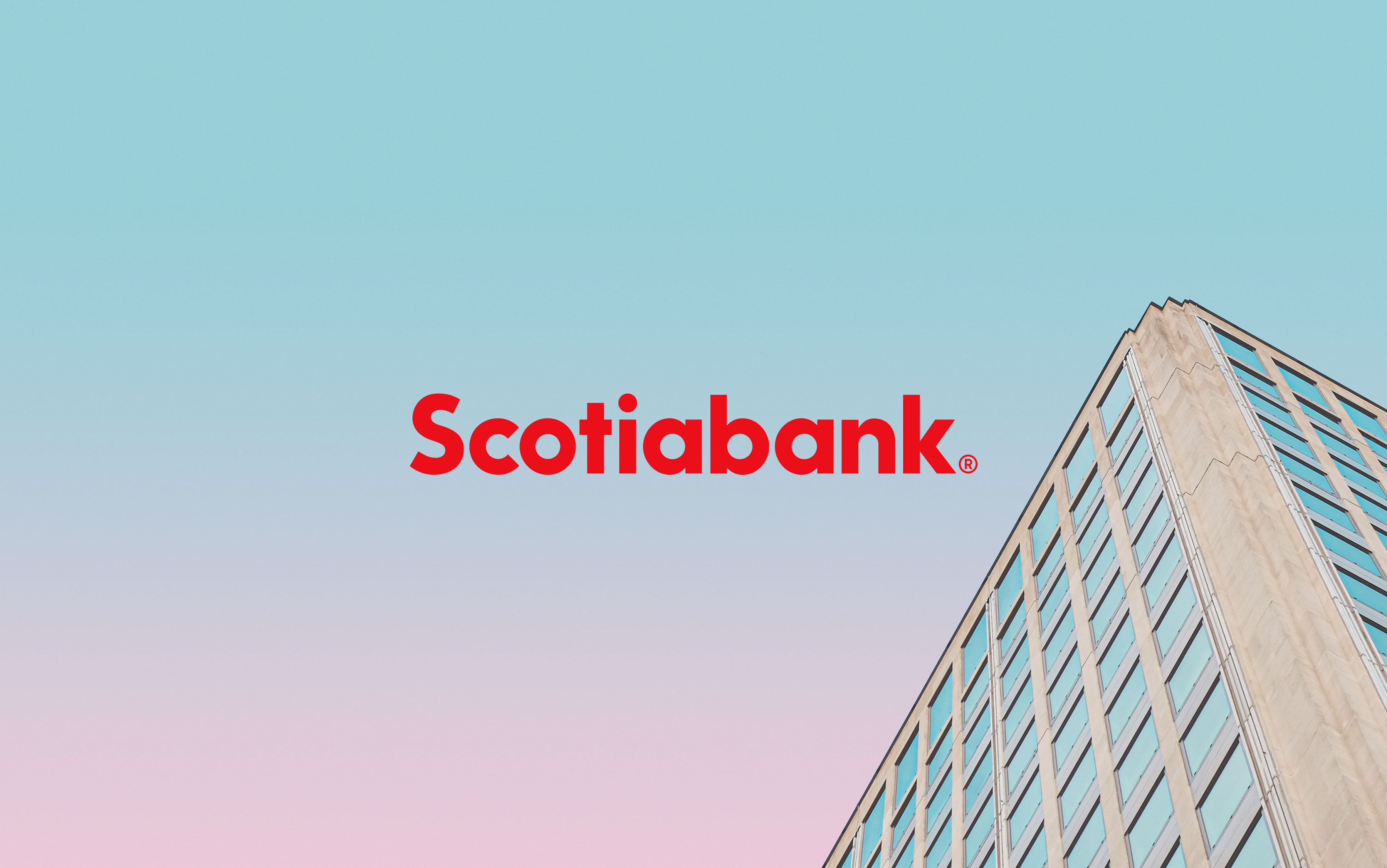
Scotiabank Taps NVIDIA AI to Improve Credit Risk Assessment
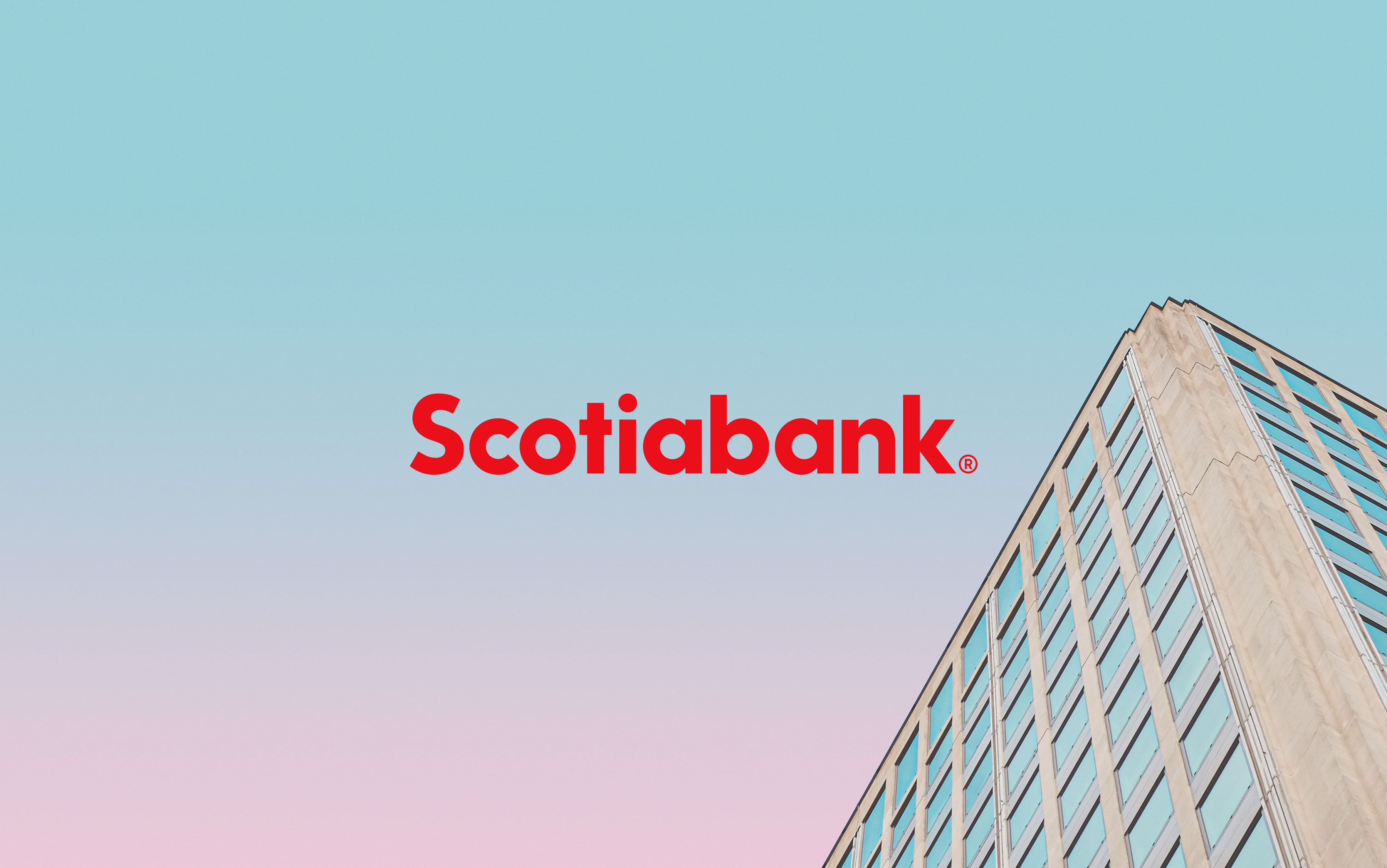
The new AI-powered method of developing credit risk scorecards is more accurate and up to six times faster.
Need to Know
- Scotiabank’s “boosting” system aims to replace traditional credit-application scorecards by creating a more accurate, yet easily-explained method of credit risk assessment.
- The boosting method is driven by machine learning and starts with a single question, adding one question at a time, then stops when additional questions would not improve results, vs. traditional scorecards with static lists of questions.
- So far, the results have been “no harder to explain than traditional weight-of-evidence models, but often were more accurate.”
- The bank will leverage NVIDIA’s AI tools to use this boosting technique to quickly generate scorecards (up to six times faster) that will give applicants credit when they are deserving of it.
Analysis
Scotiabank has adopted a new AI-driven program, in partnership with NVIDIA, that will allow credit applications to be more accurately processed.
In a blog post published on September 23, John Ashley, GM of financial services and technology at NVIDIA, detailed how the bank has updated the traditional credit-application process. Previously, the process involved a series of set, static questions issued towards a prospective lender via a scorecard. Once an applicant’s score passes a certain threshold on those scorecards, credit can be issued.
This process was earmarked as the gold standard in lending due to the fact that it can easily be explained to customers. However, this system has been in place since the 1950s and needed improvement.
Ashley wrote in his blog post that this existing system was unable to dynamically predict credit risk, as it issued identical criteria to each applicant, regardless of any nuance in their financial history.
“So, the team looked for fresh ways to build scorecards with machine learning and found a technique called boosting,” he wrote. The boosting system started with a single question, then added other questions one at a time until the AI recognized than any additional queries would not have any significant impact on an applicant’s score. There would also be no more questions once the process became to complex to be explained, ensuring both advisors and customers could understand the process.
Paul Edwards, a data scientist for Scotiabank, led his team towards the use of a library called XGBoost on an NVIDIA DGX system; this library facilitated the AI-led creation of digital scorecards. Working with NVIDIA data scientists, Scotiabank has now begun using a GPU-accelerated code within XGBoost in RAPIDS, which is “a suite of open-source software libraries for running data science on GPUs.”
“We’ve used boosting to build a couple decision models and found a few percent improvement over weight of evidence. A few percent at the scale of all the bank’s applicants means millions of dollars,” says Edwards.
Scotiabank can now generate scorecards using this system six times faster than they could before the creation of the GPU-accelerated code—and, in turn, the bank is able to more accurately serve its credit-seeking customers.
“With a much bigger pool to choose from, banks could select scorecards for their accuracy, simplicity, stability, or a balance of all these factors,” Ashley wrote. “This helps banks ensure their lending decisions are clear and reliable and that good customers get the loans they need.”
Scotiabank’s adoption of an AI model to assess credit risk follows industry trends: according to PYMNTS, of the banks that currently use AI, 71% use it for credit underwriting, while 62% of banks overall believe AI is useful for assessing credit risk.