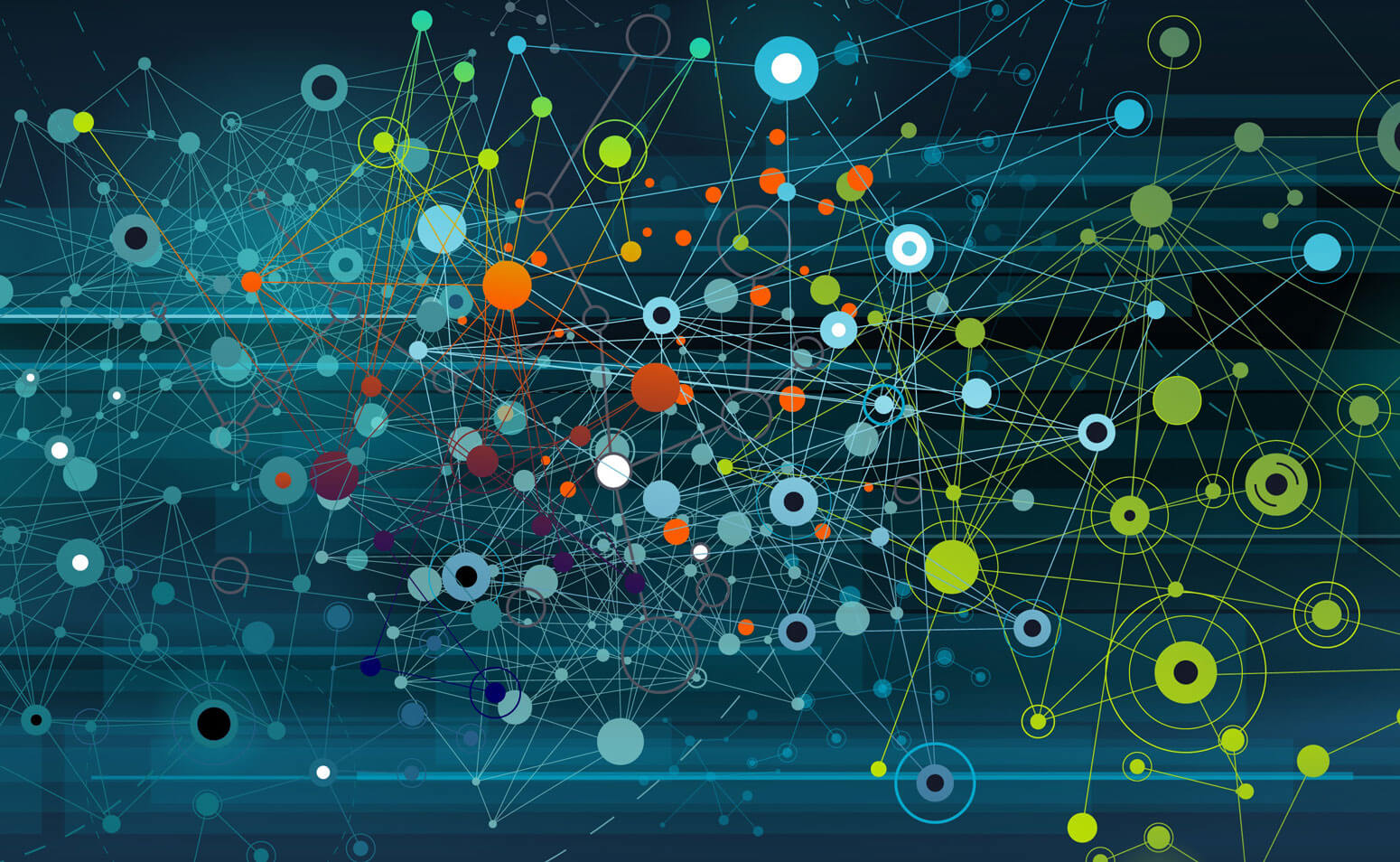
The Integral Cross-Discipline Approach to Pushing AI Research
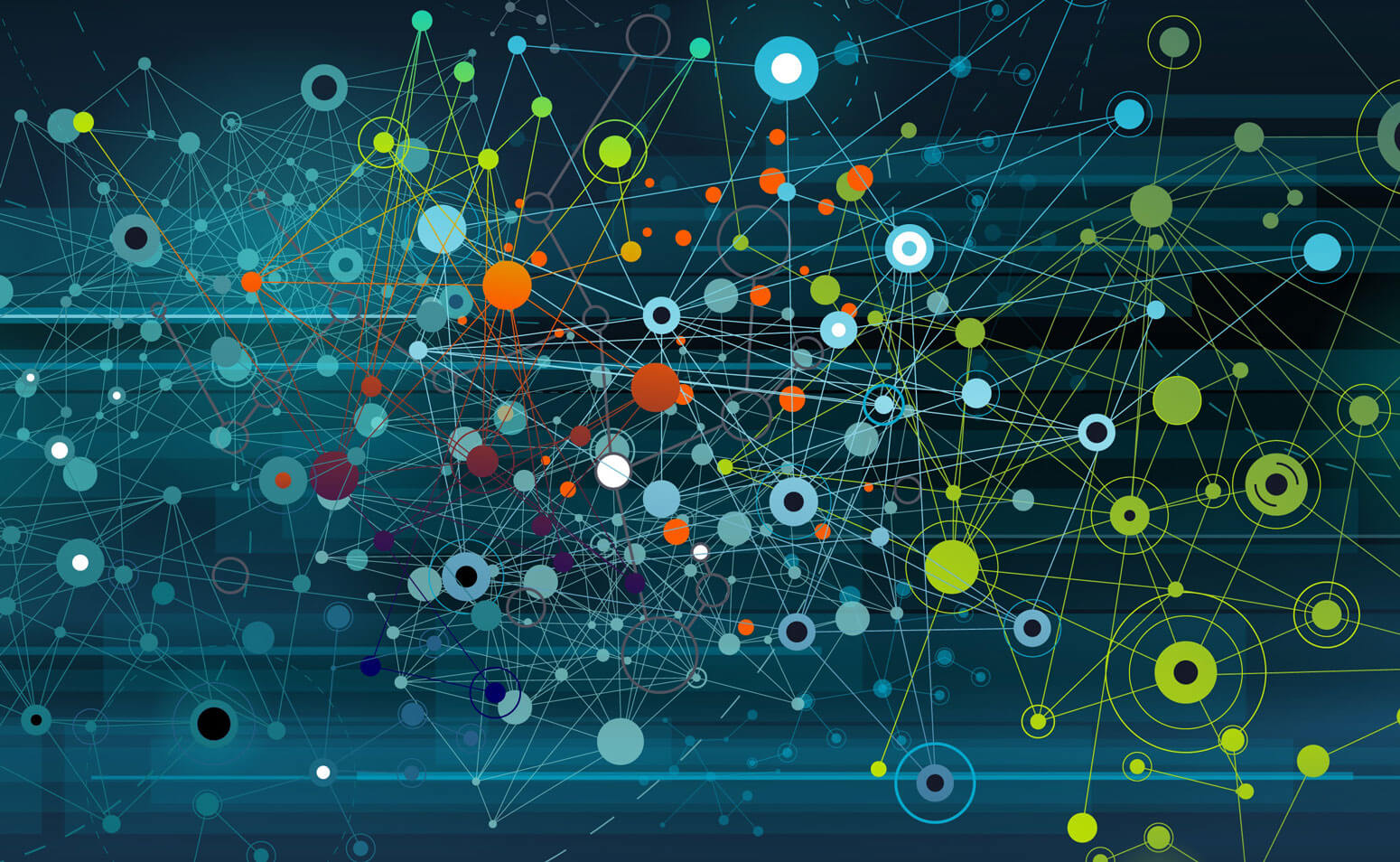
In October 2017, the Royal Swedish Academy of Sciences announced the winners of the 2017 Nobel Prize in Chemistry. The award went to Jacques Dubochet, Joachim Frank and Richard Henderson for their work in developing cryo-electron microscopy (cryo-EM), a technology that has advanced the high-resolution structure determination of biomolecules in solution.
For most researchers in artificial intelligence (AI), this award presumably failed to register. It was, after all, about a relatively new technique for the study of structural biology, which seems quite unrelated to any paper you’re likely to see at the next big AI conference. However, I would argue that this award should cause computer scientists to stand up and take notice of the potential impact of their work.
Cryo-EM involves imaging frozen samples in a transmission electron microscope and using the resulting 2D imagery to estimate the 3D structure of biomolecules. While the output of this process is of biological interest, the process itself is fundamentally computational in nature and touches on many areas of machine learning, computer vision and, more broadly, computer science. It was his contribution to the development of these methods that ultimately won Joachim Frank his portion of the Nobel Prize.
The development of cryo-EM and its recent progress is currently reshaping biologists’ ability to study life at a cellular level, an outcome that is providing the field with dramatic new insights. The direct impact of these advancements can’t be overstated. Recent results have helped researchers better understand antibiotic resistance, the mechanisms behind Alzheimer’s, and the behaviour of the Zika virus—and that’s just a small sample. As a result of this new technique, biochemistry is currently experiencing an explosion and the pharmaceutical industry is only just beginning to leverage this powerful technology to develop new life-saving treatments.
I’ve worked on computational aspects of cryo-EM for almost a decade and it’s taught me many lessons: humility while facing the difficulty of new problems, the importance of collaboration and, perhaps most importantly, the potential impact that computer scientists can have when they venture outside their comfort zone.
I was trained in computer vision and machine learning. My last biology course was in high school. Cryo-EM wasn’t an easy or intuitive problem for me and, when I started, it wasn’t clear that it would be successful. In fact, the only thing that was clear is that if it succeeded, the potential impact would be far greater than anything I’d ever attempted before.
In recent years, there have been dramatic advances in computer science—specifically in machine learning and artificial intelligence—and we are only starting to scratch the surface of what’s possible. Cryo-EM is just one of many applications with a potentially transformative impact on the world, and these applications require computational expertise and the development of new techniques.
It’s by recognizing the sheer breadth of possibility in these applications that I’ve developed a new perspective: As researchers and practitioners, it’s ultimately up to us to decide how these techniques are applied and to prioritize which applications are most important. We make these decisions and set these priorities with each new project we undertake and, because of the massive responsibility these judgment calls entail, I believe it’s now more important than ever to question the value and societal impact of the work we do.
So, every time a researcher and practitioner considers their next project, I challenge them to ask themselves a simple question: Is this really the most impact you can have? If it’s not, then keep pushing the boundaries.
Marcus Brubaker is a professor at York University and a senior researcher at the Borealis AI institute in Toronto. He specializes in Bayesian machine learning and cryo-EM.